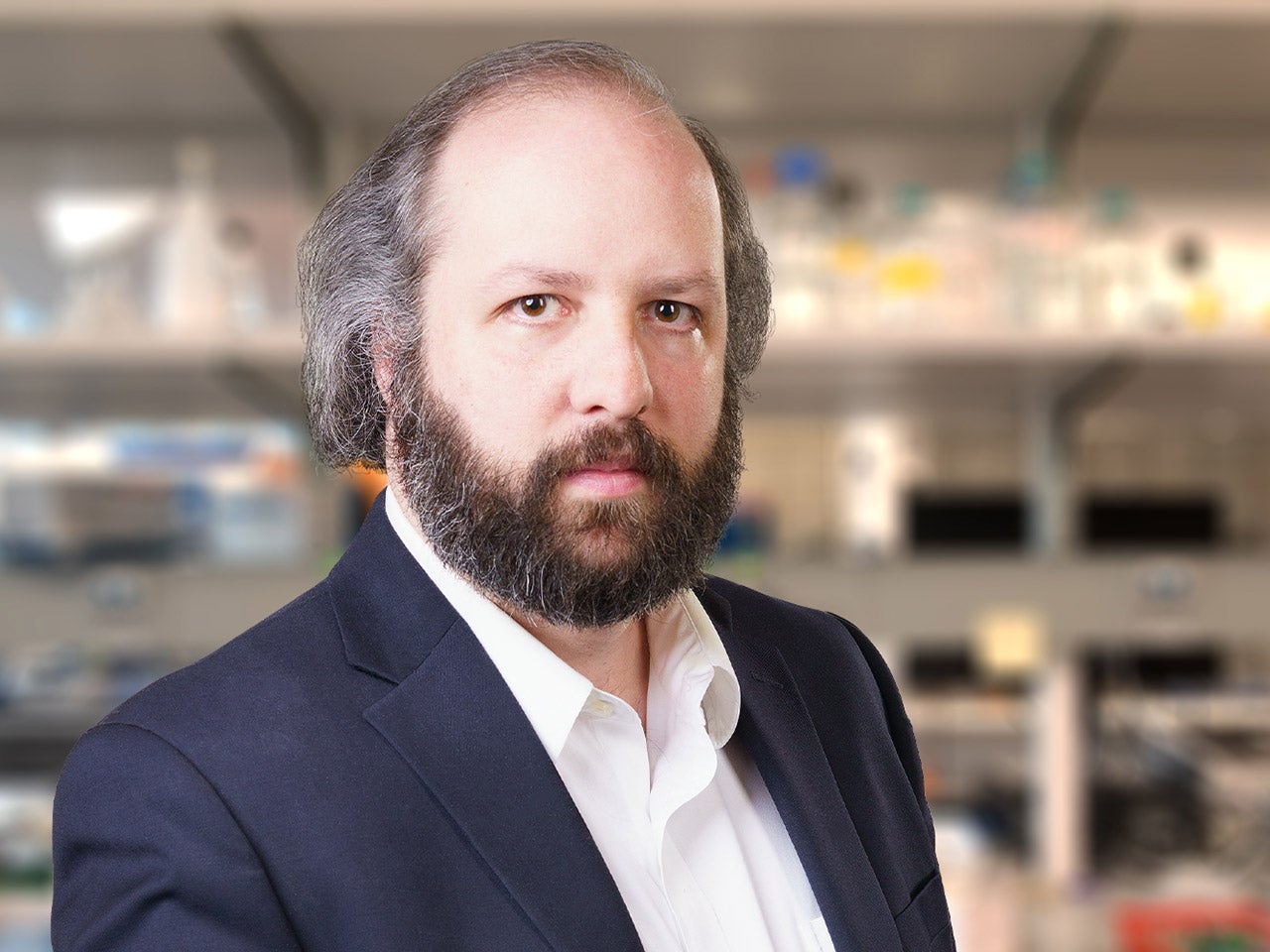
David McCandlish
Associate Professor
Cancer Center Member
Ph.D., Duke University, 2012
mccandlish@cshl.edu | 516-367-5286
Faculty ProfileSome mutations are harmful but others are benign. How can we predict the effects of mutations, both singly and in combination? Using data from experiments that simultaneously measure the effects of thousands of mutations, I develop computational tools to predict the functional impact of mutations and apply these tools to problems in protein design, molecular evolution, and cancer.
The McCandlish lab develops computational and mathematical tools to analyze and exploit data from high-throughput functional assays. The current focus of the lab is on analyzing data from so-called “deep mutational scanning” experiments. These experiments simultaneously determine, for a single protein, the functional effects of thousands of mutations. By aggregating information across the proteins assayed using this technique, we seek to develop data-driven insights into basic protein biology, improved models of molecular evolution, and more accurate methods for predicting the functional effects of mutations in human genome sequences.
Critically, these data also show that the functional effects of mutations often depend on which other mutations are present in the sequence. We are developing new techniques in statistics and machine learning to infer and interpret the complex patterns of genetic interaction observed in these experiments. Our ultimate goal is to be able to model these sequence-function relationships with sufficient accuracy to guide the construction of a new generation of designed enzymes and drugs, and to be able to predict the evolution of drug resistance phenotypes in both populations of cancer cells and rapidly evolving microbial pathogens.
You say genome editing, I say natural mutation
October 19, 2023
CSHL scientists have discovered that evolution and genome editing in crops are less predictable than previously thought.
How evolved is your knowledge?
January 26, 2023
Test your knowledge of evolution with this quiz, inspired by the March 2023 performances of Isabella Rossellini’s play, Darwin’s Smile, at CSHL.
Getting a step ahead of TB’s drug resistance evolution
February 15, 2022
Mutations are not random, with some kinds of changes occurring more often than others. CSHL researchers may be able to predict which direction evolution is li
Calculating the path of cancer
October 4, 2021
A new mathematical approach is helping cancer researchers at CSHL determine how mutations lead to different behaviors in cancerous cells.
How to outwit evolution
July 21, 2021
CSHL Assistant Professor David McCandlish uses statistical methods to predict the evolution of antibiotic resistance in bacteria.
Predicting the evolution of genetic mutations
April 14, 2020
CSHL quantitative biologists have designed a computational approach for predicting the evolution of a rapidly mutating virus or cancer.
A science career path: David McCandlish
April 10, 2020
Assistant Professor David McCandlish is a quantitative biologist who walks the line between advanced mathematics and the life sciences at CSHL.
Peter Koo wants to understand how machines learn biology
September 20, 2019
Dr. Peter Koo joins the CSHL faculty as an assistant professor. His focus is on exploring how artificial intelligence integrates with biology and genomics.
Event: Public Lecture: Seeing With Sequencing
August 8, 2019
Come hear from three quantitative biologists as they discuss how they see with sequencing to solve mysteries ranging from the genetics of evolution.
Seeing with sequencing—A public lecture with three CSHL experts
April 19, 2019
Quantitative biologists discuss how physics, modern computing power, and a new perspective on biology can make sense of our complex genomes.
All Publications
Guidelines for releasing a variant effect predictor
15 Apr 2025 | Genome Biology | 26(1):97
Livesey, Benjamin; Badonyi, Mihaly; Dias, Mafalda; Frazer, Jonathan; Kumar, Sushant; Lindorff-Larsen, Kresten; McCandlish, David; Orenbuch, Rose; Shearer, Courtney; Muffley, Lara; Foreman, Julia; Glazer, Andrew; Lehner, Ben; Marks, Debora; Roth, Frederick; Rubin, Alan; Starita, Lea; Marsh, Joseph;  
Evolutionary paths that link orthogonal pairs of binding proteins
10 Apr 2025 | Protein Science | 32(12):101262
Avizemer, Ziv; Martí-Gómez, Carlos; Hoch, Shlomo; McCandlish, David; Fleishman, Sarel;  
Symmetry, gauge freedoms, and the interpretability of sequence-function relationships
1 Apr 2025 | Physical Review Research | 7(2)
Posfai, A; McCandlish, D; Kinney, J;  
Gauge fixing for sequence-function relationships
20 Mar 2025 | PLoS Computational Biology | 21(3):e1012818
Posfai, Anna; Zhou, Juannan; McCandlish, David; Kinney, Justin; Patil, Kiran;  
Inference and visualization of complex genotype-phenotype maps with gpmap-tools
13 Mar 2025 | bioRxiv
Martí-Gómez, Carlos; Zhou, Juannan; Chen, Wei-Chia; Kinney, Justin; McCandlish, David;  
Cryptic variation fuels plant phenotypic change through hierarchical epistasis
25 Feb 2025 | bioRxiv
Zebell, Sophia; Martí-Gómez, Carlos; Fitzgerald, Blaine; Pinto Da Cunha, Camila; Lach, Michael; Seman, Brooke; Hendelman, Anat; Sretenovic, Simon; Qi, Yiping; Bartlett, Madelaine; Eshed, Yuval; McCandlish, David; Lippman, Zachary;  
Density estimation for ordinal biological sequences and its applications
30 Oct 2024 | Physical Review E | 110(4)
Chen, Wei-Chia; Zhou, Juannan; McCandlish, David;  
A fitness distribution law for amino-acid replacements
15 Oct 2024 | bioRxiv
Sun, Mengyi; Stoltzfus, Arlin; McCandlish, David;  
A side-by-side comparison of variant function measurements using deep mutational scanning and base editing
26 Sep 2024 | bioRxiv
Sokirniy, Ivan; Inam, Haider; Tomaszkiewicz, Marta; Reynolds, Joshua; McCandlish, David; Pritchard, Justin;  
Multiple distinct evolutionary mechanisms govern the dynamics of selfish mitochondrial genomes in Caenorhabditis elegans
19 Sep 2024 | Nature Communications | 15(1):8237
Gitschlag, Bryan; Pereira, Claudia; Held, James; McCandlish, David; Patel, Maulik;