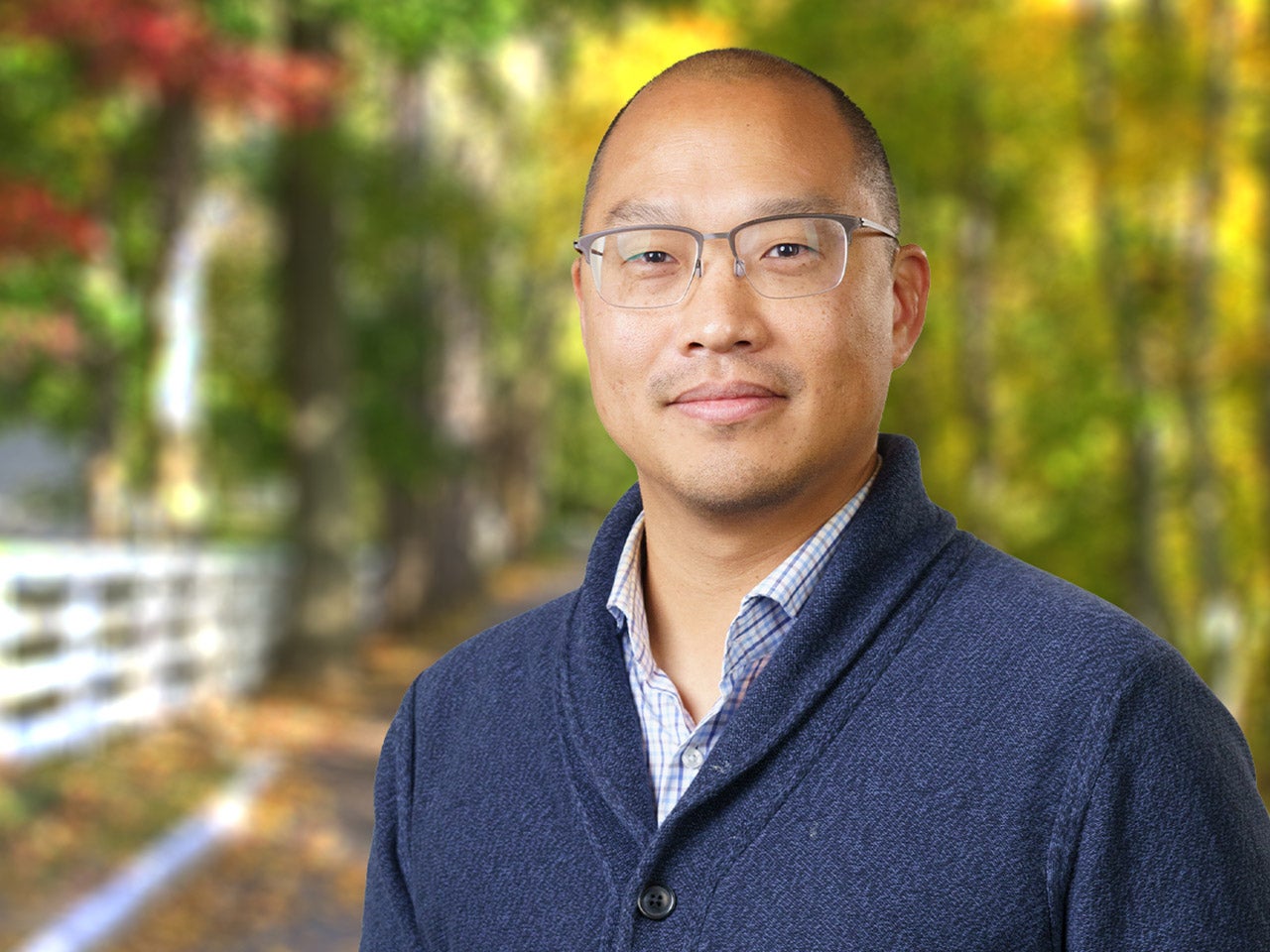
Peter Koo
Associate Professor
Cancer Center Member
Ph.D., Yale University, 2015
koo@cshl.edu | 516-367-5520
Deep learning has the potential to make a significant impact in basic biology and cancer, but a major challenge is understanding the reasons behind their predictions. My research develops methods to interpret this powerful class of black box models, with a goal of elucidating data-driven insights into the underlying mechanisms of sequence-function relationships.
Deep learning is being applied rapidly in many areas of genomics, demonstrating improved performance over previous methods on benchmark datasets. Despite the promise of deep learning, it remains unclear whether improved predictions will translate to new biological discoveries because of their low interpretability, which has earned them a reputation as a black box. Understanding the reasons underlying a deep learning model’s prediction may reveal new biological insights not captured by previous methods. Our group develops methods to interpret high-performing deep learning models to distill knowledge that they learn from big, noisy, biological sequence data. Our goal is to elucidate biological mechanisms that underlie sequence-function relationships for gene regulation and protein (dys)function. Recently, we have teamed up with other members of the CSHL Cancer Center to investigate the sequence basis of epigenomic differences across healthy and cancer cells.
At the Lab Season 1 Research Rewind: AI+
October 29, 2024
This season’s final Research Rewind brings us from the realm of quantitative biology to neuroscience, genomics, and beyond.
CSHL grad student wins International Birnstiel Award
September 23, 2024
Shushan Toneyan won the award for her thesis research in CSHL’s Koo lab. Toneyan is the co-creator of CREME, an AI-powered virtual laboratory.
Is CREME AI’s answer to CRISPR?
September 16, 2024
CREME, the latest AI toolkit from CSHL, is a virtual laboratory that may help scientists find new therapeutic targets in the genome.
At the Lab Episode 17: AI SQUID
July 30, 2024
Tune in to this week’s podcast to hear about the latest artificial intelligence model coming out of Cold Spring Harbor Laboratory.
SQUID pries open AI black box
June 21, 2024
CSHL’s Koo and Kinney labs have built a tool to suss out how AI analyzes the genome. What sets it apart? Decades of quantitative genetics knowledge.
The CSHL School of Biological Sciences’ class of 2024
May 5, 2024
The School of Biological Sciences awarded Ph.D. degrees to 11 students this year. Here are some stories and reflections from their time at CSHL.
Can AI uncover breast cancer risk factors?
February 26, 2024
This question lies at the heart of a new interdisciplinary collaboration between CSHL’s Camila dos Santos and Peter Koo.
The digital dark matter clouding AI
June 5, 2023
Scientists have unknowingly encountered mysterious noise while using AI to decipher our genetic code. CSHL has found a way to cut through the fog.
AI training: A backward cat pic is still a cat pic
May 4, 2023
This basic rule of thumb is helping CSHL’s quantitative biologists train AI to get a better read of the human genome.
Can you outsmart this AI quiz?
February 6, 2023
Think you’re plugged into the latest artificial intelligence advancements? Test your tech knowledge with this quiz on AI and computational biology.
All Publications
Machine Learning for Protein Science and Engineering
5 May 2025 | Cold Spring Harbor Perspectives in Biology | :a041877
Koo, Peter; Dallago, Christian; Nambiar, Ananthan; Yang, Kevin;  
Massive experimental quantification allows interpretable deep learning of protein aggregation
2 May 2025 | Science Advances | 11(18):eadt5111
Thompson, Mike; Martín, Mariano; Olmo, Trinidad; Rajesh, Chandana; Koo, Peter; Bolognesi, Benedetta; Lehner, Ben;  
Probabilistic and machine-learning methods for predicting local rates of transcription elongation from nascent RNA sequencing data
8 Feb 2025 | Nucleic Acids Research (NAR) | 53(4)
Liu, Lingjie; Zhao, Yixin; Hassett, Rebecca; Toneyan, Shushan; Koo, Peter; Siepel, Adam;  
Probabilistic and machine-learning methods for predicting local rates of transcription elongation from nascent RNA sequencing data.
8 Feb 2025 | Nucleic Acids Research (NAR) | 53(4)
Liu, Lingjie; Zhao, Yixin; Hassett, Rebecca; Toneyan, Shushan; Koo, Peter; Siepel, Adam;  
Reusable specimen-level inference in computational pathology
10 Jan 2025
Kaczmarzyk, Jakub; Sharma, Rishul; Koo, Peter; Saltz, Joel;  
Uncertainty-aware genomic deep learning with knowledge distillation
15 Nov 2024 | bioRxiv
Zhou, Jessica; Rizzo, Kaeli; Tang, Ziqi; Koo, Peter;  
A community effort to optimize sequence-based deep learning models of gene regulation
11 Oct 2024 | Nature Biotechnology
Rafi, Abdul; Nogina, Daria; Penzar, Dmitry; Lee, Dohoon; Lee, Danyeong; Kim, Nayeon; Kim, Sangyeup; Kim, Dohyeon; Shin, Yeojin; Kwak, Il-Youp; Meshcheryakov, Georgy; Lando, Andrey; Zinkevich, Arsenii; Kim, Byeong-Chan; Lee, Juhyun; Kang, Taein; Vaishnav, Eeshit; Yadollahpour, Payman; Random Promoter DREAM Challenge Consortium; Kim, Sun; Albrecht, Jake; Regev, Aviv; Gong, Wuming; Kulakovskiy, Ivan; Meyer, Pablo; de Boer, Carl;  
Analysis of single-cell CRISPR perturbations indicates that enhancers predominantly act multiplicatively
10 Oct 2024 | Cell Genomics | :100672
Zhou, Jessica; Guruvayurappan, Karthik; Toneyan, Shushan; Chen, Hsiuyi; Chen, Aaron; Koo, Peter; McVicker, Graham;  
Interpreting cis-regulatory interactions from large-scale deep neural networks
16 Sep 2024 | Nature Genetics
Toneyan, Shushan; Koo, Peter;  
Explainable AI for computational pathology identifies model limitations and tissue biomarkers
4 Sep 2024
Kaczmarzyk, Jakub; Koo, Peter; Saltz, Joel;